

These articles were kept separate until we had final models to test, therefore these articles were only used for final testing and performance evaluation. Qualifying articles published in 20 made up the evaluation test data sets. This data set was used for initial testing and software verification, and also used to improve model calibration using a novel training and re-calibration approach that will be described later. All qualifying PubMed articles published in 2015 made up the validation data set.

This data set was used to select features, models, and algorithms. All qualifying PubMed articles published between 1987-2014 made up the training data set.
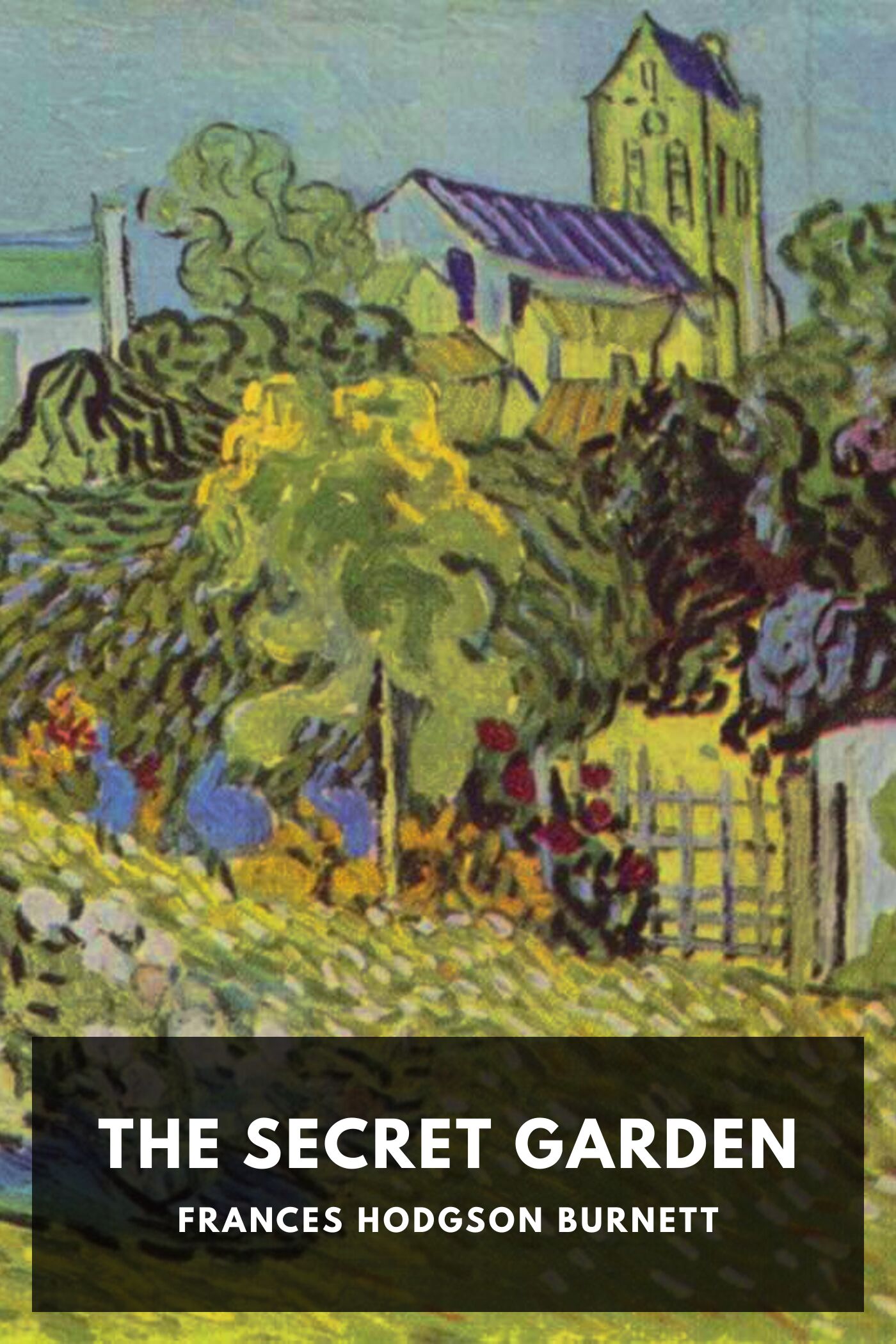
To train, calibrate, and test the set of probabilistic tagger machine learning models, separate training, validation, and evaluation datasets were created, consisting of PubMed articles having abstracts and written in English (or, if not written in English, having English abstracts), which were human-related (i.e., were indexed with the Humans MeSH term). In the work presented here, a set of 50 probabilistic machine-learning based taggers have been developed and evaluated for a wide range of publication types and study designs, and applied to the entire PubMed indexed literature. In previous work, we have proposed the use of automated assignment of probability confidence-based tags which provide a predictive score between 0.0 and 1.0 for randomized controlled trials and human-related studies created by machine learning models. Second, we do not attempt to index an article according to its “best” few MeSH terms, but rather consider each possible PT on its own merits, and attempt to estimate the probability that the article can be regarded as belonging to that PT. First, in the present study, we focus only on those MeSH terms which refer to Publication Types (e.g., review, case report, news article, etc.) and clinically relevant study designs (e.g., case-control study, retrospective study, random allocation, etc.), both abbreviated here as PTs. Here our goal is also to create an automated MeSH indexing scheme for biomedical literature, but our approach differs in several important respects. Other tools such as RobotAnalyst, Abstracker, SWIFT-Review ( ), and EPPI reviewer, incorporate machine learning processes to reduce the need for manual review based on initial groups of manual judgements. Tools such as RCT Tagger and Robotsearch allow identifying articles that are likely to be randomized controlled trials. Previous work, by our team and others, has focused on using machine learning to rank or filter articles for inclusion in systematic reviews. MeSHProbeNet builds on these approaches, improving the time and space efficiency by creating fixed dimensional context vectors and predicting all MeSH terms simultaneously. FullMeSH has enhanced these approaches by applying section-based convolutional neural networks to full article text.

More recently, a combined MeSHLabeler and DeepMeSH system used learning to rank along with Deep Learning semantic representation to achieve the highest binary prediction scores in the BioASQ2 and BioASQ3 challenges. built a MeSH term recommender system that used PubMed Related Articles and a learning-to-rank approach. For example, the National Library of Medicine’s Medical Text Indexer (MTI) predicted a set of MeSH terms to be used as suggestions to a manual indexer. Previous work on automated MeSH indexing of publications has primarily focused on predicting a small set of top or “best” set of MeSH terms for a given article. Only a subset of MeSH headings refer to study designs or allocation schemes such as case-control, cross-over, and randomized clinical trials. One way to retrieve or filter literature is based on their indexing according to Medical Subject Headings (MeSH). Filtering literature based on study designs can provide substantial benefit early in the systematic review process. New computer-based tools and approaches are required to keep up with the ever-expanding biomedical literature base. Identifying appropriate articles involves time consuming searching and manual review. The biomedical literature is often used to retrieve and synthesize knowledge for uses such as evidence-based medicine, systematic review, meta-analysis, practice guidelines, narrative reviews, and knowledge discovery.
